(Bloomberg Opinion) -- The analysts at Goldman Sachs Group Inc. are at it again — trying to predict soccer outcomes and getting it all wrong. Far be it from me to hurl brickbats, however: This repeated exercise in analytical courage shows to all of us in the data business, and to those on the receiving end of it, that even after a sophisticated model has been proven faulty and refactored, the real world can quickly make a mockery of it again.
Goldman’s prognostications on the football pitch are not a trivial enterprise. People make lots of money with complex financial market models; AI models beat humans at all kinds of games, from poker to Go; and Michael Lewis’s 2003 book, “Moneyball: The Art of Winning an Unfair Game,” and the excellent movie based on it describe the successful application of statistical modeling to baseball. Soccer, however, is unlike any of these environments in that it’s even more chaotic and difficult to formalize. Its randomness, the abundance of errors, the scarcity of spectacular results, the undeniable role played by sheer luck — all these things make it, in the end, a lot like life, which I suspect is at least part of the secret behind its enduring popularity.
So as more people are tempted to trust data-based models, which keep getting better as technology develops, with real-life decisions or at least meaningful contributions to, say, pandemic-related policies, they ought to give some thought to the Goldman experiments with predicting soccer. Thanks to the willingness of the analysts behind them — above all Sven Jari Stehn, who has taken part in the 2014, 2018 and 2021 exercises — to be publicly wrong, not something analysts and modelers generally enjoy, we can all see that experience and improvements in technological tools aren’t yet enough to take on a truly complex system.
I wrote before about the spectacularly wrong predictions by the 2014 and 2018 models, made despite the analysts’ determination to learn from their mistakes and add more potentially important factors to their analysis. The desire to improve was also evident in the paper describing Goldman’s approach to Euro 2020, the continental championship being played a year late because of the Covid-19 pandemic. After giving the 2018 World Cup to Brazil, which was supposed to beat Germany in the final game (France beat Croatia instead), the analysts decided to stop messing around with player-level statistics and go instead with team performance and rankings, especially since these are highly correlated with player-level indicators such as transfer values. Instead of victory probabilities as before, the current version of the model tries to predict the number of goals each team can score against its rivals. In a way, it’s the “Moneyball” approach of focusing on the key statistic for success.
The analysts wrote:
We start by modelling the number of goals scored by each team using a large dataset of international football matches since 1980. We find that the number of goals scored by each team can be explained by (1) the strength of the squad (measured with the World Football Elo Rating), (2) goals scored and conceded in recent games (capturing the side’s momentum), (3) home advantage (which is worth 0.4 goals per game) and (4) a tournament effect (which shows that some countries tend to outperform at tournaments compared to their rating).
In other words, the current model builds on previous experience and distills the many statistics one can analyze in soccer to a set of metrics most significant for final scores.
And yet the very first days of the tournament have shown that this new approach works no better than previous ones.
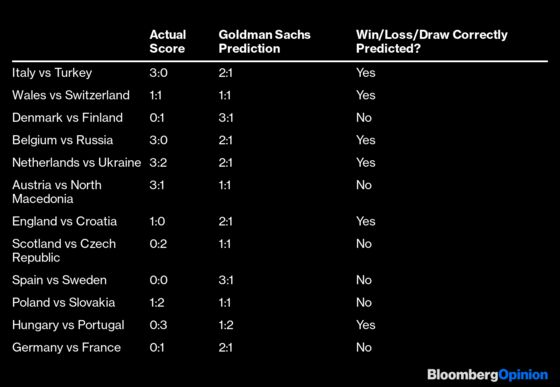
It would be unfair to demand from Goldman Sachs that it correctly predict the actual scores. But out of the first 12 games, it only got six outcomes right. Such abysmal performance cannot be written down to random events like the sudden collapse of Danish star Christian Eriksen during the game with Finland. The heart stoppage, which Eriksen survived, probably cost his stricken teammates the victory Denmark’s superior record promised. Most of the other games, however, weren’t eventful in that way — the randomness level was mostly normal. If anything, the randomness helped Goldman get its few wins: Hungary, for example, looked about to play Portugal to a draw in the packed Budapest stadium — but it suddenly came unstuck in the final 10 minutes of the game, conceding with a much higher score than the first 80 minutes warranted.
Before the Germany-France game in Munich, Stehn conceded that the model must be overestimating the home advantage factor. “Speaking as a German,” he added, “the home advantage hopefully makes a comeback.” Germany, of course, lost the home game, defying another Goldman prediction. An own goal by 2014 world champion Mats Hummels won the day for France — show me the model that could have predicted something like this — but France was also clearly the better team, scoring two goals that were disallowed on the offsides rule but that demonstrated the current world champion’s speed and creativity advantage.
Given all this, I don’t really know what to make of Goldman’s prediction that Belgium, currently the world’s highest-ranked team, will win the tournament, defeating Portugal in the final. Betting on the top-ranked contender is generally safer than picking an outsider — but, firstly, one doesn’t need a complex model to make that decision, and secondly, it doesn’t look as though Goldman, with all its previous soccer modeling experience, has settled on the right set of data points for its model.
In this data-obsessed world with its increasingly perfect measuring tools, we get every kind of metric live during a soccer game. UEFA, European soccer’s governing body, provides a slew of statistics, both team and individual. It’ll be interesting to see at the end of the tournament how all of them correlate with final results — but even the most obvious of them can contrast starkly with the outcome of a specific game. Spain spent 86% of the time in possession of the ball versus Sweden; its players made 917 passes with 90% accuracy, compared with just 162 with 54% accuracy for their rivals. The score? A 0:0 draw.
In a game where every match has an individual character thanks to a signature combination of factors, a confluence of random and predetermined events, a clash of several dozen individual temperaments and several coaches’ attempts to bring order to the chaos, statistical regularities can still work in the long run. But tournaments are short-run events, their outcome dependent on these individual games, arguably to a greater extent than on long-term tendencies. That makes picking the relevant data extremely hard, if not completely pointless.
But then, such is life, too. One could build infection-minimizing models during peak Covid based on its first wave and on previous pandemics — but they were constantly waylaid by new virus mutations, inaccurate predictions of vaccine deliveries and vaccination progress, varying reactions to contact restrictions and every other imaginable kind of “black swan.” I’d be hard put to name an expert who has accurately predicted the timeline of the pandemic’s ebbs and flows; the only wisdom I can derive from watching the attempts at data-based policy-making is, “Always prepare for the worst.” That’s just about as powerful an insight as “Bet on the top-ranking team.”
Modeling is an exercise that makes those of us who practice it feel exceedingly smart. There’s a strong temptation not to be put off by the facts if they suddenly begin to contradict a model: One can always keep waiting for a long enough horizon. So I’m grateful to the highly competent Goldman analysts who make a regular spectacle of themselves with their soccer models: This is the kind of cold shower the “information age” needs even more regularly than the major soccer events that are held and mispredicted.
This column does not necessarily reflect the opinion of the editorial board or Bloomberg LP and its owners.
Leonid Bershidsky is a member of the Bloomberg News Automation team based in Berlin. He was previously Bloomberg Opinion's Europe columnist. He recently authored a Russian translation of George Orwell's "1984."
©2021 Bloomberg L.P.