The Credit Robot at HSBC Says Odds of a Bear Market Are Now 84%
The Credit Robot at HSBC Says Odds of a Bear Market Are Now 84%
(Bloomberg) -- From booming bond sales and benign default rates to benevolent central banks, all seems well in the credit market right now. That’s unless you ask the robot prognosticator at HSBC Holdings Plc.
The machine-learning model reckons there’s now an 84% chance of a bear market sweeping U.S. corporate debt within the next year. That’s the highest level since before the financial crisis and “a validation” of HSBC’s mildly bearish view, according to strategists led by Song Jin Lee.
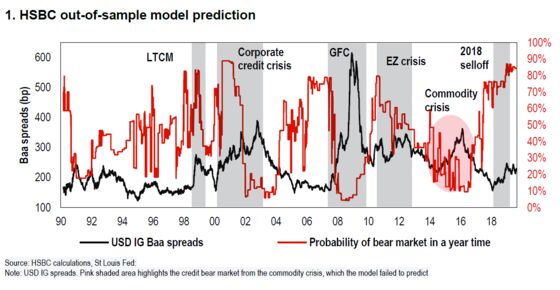
“This suggests the year-to-date rally in credit spreads is likely to be a temporary ‘pullback’ before the big sell-off hits in 2020,” the team wrote in new research this month.
The urgent question for investors is what the robot knows that the market doesn’t.
HSBC’s credit strategists are among the swelling cohort of investment banks and investors around the globe who are complementing their forecasts with this much-touted branch of artificial intelligence, which seeks to perform cutting edge data analysis without human guidance at every step.
The bearish-credit robot uses an advanced version of a decision tree -- geeks would call it an XGBoost model -- to divine the chances of a market downturn from nearly a dozen indicators. The pitch is that, by learning from its mistakes and mapping the complex relationships between various factors, it can make better predictions than humans using more traditional approaches.
HSBC trained its model with four decades of data, from 1950 to 1989, then tested it on data from 1990 onward. It accurately predicted five of the last six credit bear markets with a lead time of between seven and 16 months.
To skeptics, machine-learning is often little more than a marketing exercise that provides no great advance in sorting signal from noise. And for now at least, markets show few overt signs of an imminent downturn. High-yield credit spreads have tightened this year to far below the five-year average on expectations a global return to monetary easing will drive more money into bonds and avert a recession along the way.
HSBC acknowledges these models can seem like a “black box,” but the team does delve into one process to support its predictions. Known as SHAP, it’s a machine-learning technique that essentially tries to explain predictions by simplifying the model. It shows two indicators flashing red for U.S. corporate debt now: elevated consumer confidence, which tends to precede economic weakness, and a flat Treasury yield curve.
Other inputs include things like bond issuance, earnings growth and truck sales.
Two more caveats: Some degree of human intervention is still necessary, mostly in the initial phase of gauging and improving data quality. And the model tends to miss bear markets triggered by external shocks, such as a crash in commodities.
“This exercise demonstrates the versatility of tree-based machine learning algorithms, even when faced with poor quality data and/or a large imbalance in the occurrence of the predicted state (in this case, credit bear markets),” the team said. “But as flexible as these algorithms are, they still require a helping hand from friendly humans.”
To contact the reporter on this story: Justina Lee in London at jlee1489@bloomberg.net
To contact the editors responsible for this story: Samuel Potter at spotter33@bloomberg.net, Sid Verma
©2019 Bloomberg L.P.